Statistical Analysis
Turning Complex Data into Clear, Actionable Insights
Comprehensive Analytical Approaches
- Parametric & Nonparametric Tests: We apply t-tests, ANOVAs, Mann–Whitney U, and other classical methods to measure treatment effects or differences in distribution.
- Confidence Intervals & Hypothesis Testing: Through meticulous planning (e.g., power analysis, alpha-level adjustments), we ensure robust and reproducible outcomes.
- Linear & Logistic Regression: Evaluating relationships between explanatory variables and continuous or binary outcomes, commonly used in efficacy and risk factor studies.
- Generalized Linear Models (GLMs) & Mixed-Effect Models: Addressing nested data structures, repeated measures, and random effects to handle complex clinical trial designs or longitudinal cohort data.
- Kaplan–Meier & Cox Proportional Hazards Models: Essential for oncology or chronic disease studies, these methods capture event times (e.g., time-to-death, time-to-relapse).
- Competing Risks & Time-Dependent Covariates: We handle scenarios where multiple events or changing conditions can alter the hazard function over time.
- Hierarchical Bayesian Modeling: Allows us to incorporate prior knowledge (e.g., historical controls) and produce posterior distributions that reflect uncertainty more transparently.
- Adaptive Trial Designs: Using Bayesian updating, we can adjust sample sizes, randomization ratios, or inclusion criteria mid-study, improving trial efficiency and patient safety.
- Random Forests, Gradient Boosting, Neural Networks: Helpful for large datasets from wearables, EHRs, or real-world registries. These algorithms identify complex patterns and interactions.
- Feature Engineering & Model
Validation: Rigorously evaluating performance
through cross-validation, calibration curves, and external validation sets
ensures reliability.
- Evidence Synthesis: We combine results from multiple independent studies, performing quantitative integrations (fixed-effect or random-effects models) and exploring heterogeneity for interventional (clinical trials) and non-interventional (diagnostic accuracy and observational studies) studies.
- Publication Bias & Sensitivity Analyses: Egger’s tests and funnel plots help detect biases, while subgroup and sensitivity analyses clarify data robustness.
- Budget Impact & Cost-Utility Analyses: We evaluate the economic implications of interventions, measuring outcomes like Quality-Adjusted Life Years (QALYs) and incremental cost-effectiveness ratios (ICERs).
- Decision-Analytic Modeling: Markov models or decision trees may be employed to project long-term clinical and economic impacts under various scenarios.
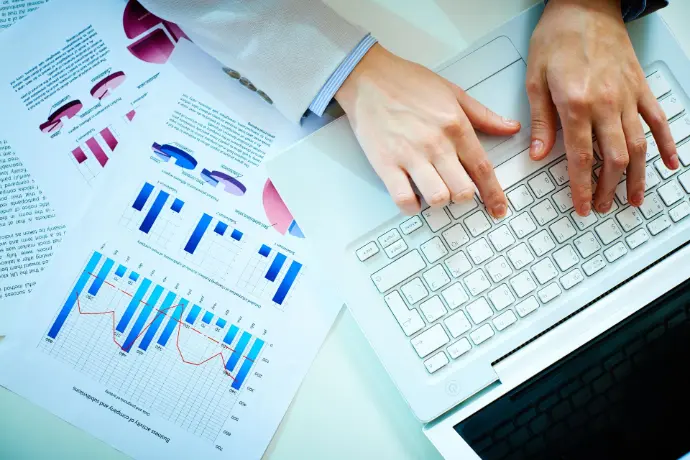
Software & Infrastructure
SAS, STATA, & and SPSS
We use these industry-standard tools for data cleaning, advanced modeling, and sophisticated statistical tests.
Open-Source & Specialized Tools
Our team is also adept with R, Python (for machine learning), and specialized Bayesian software (e.g., WinBUGS, Stan) to accommodate highly customized methodologies.
Secure, Compliant Environment
All analyses occur in an environment compliant with HIPAA, GDPR, and other pertinent data-privacy regulations, ensuring confidentiality and data integrity.
Key Benefits of Kernel’s Statistical Analysis